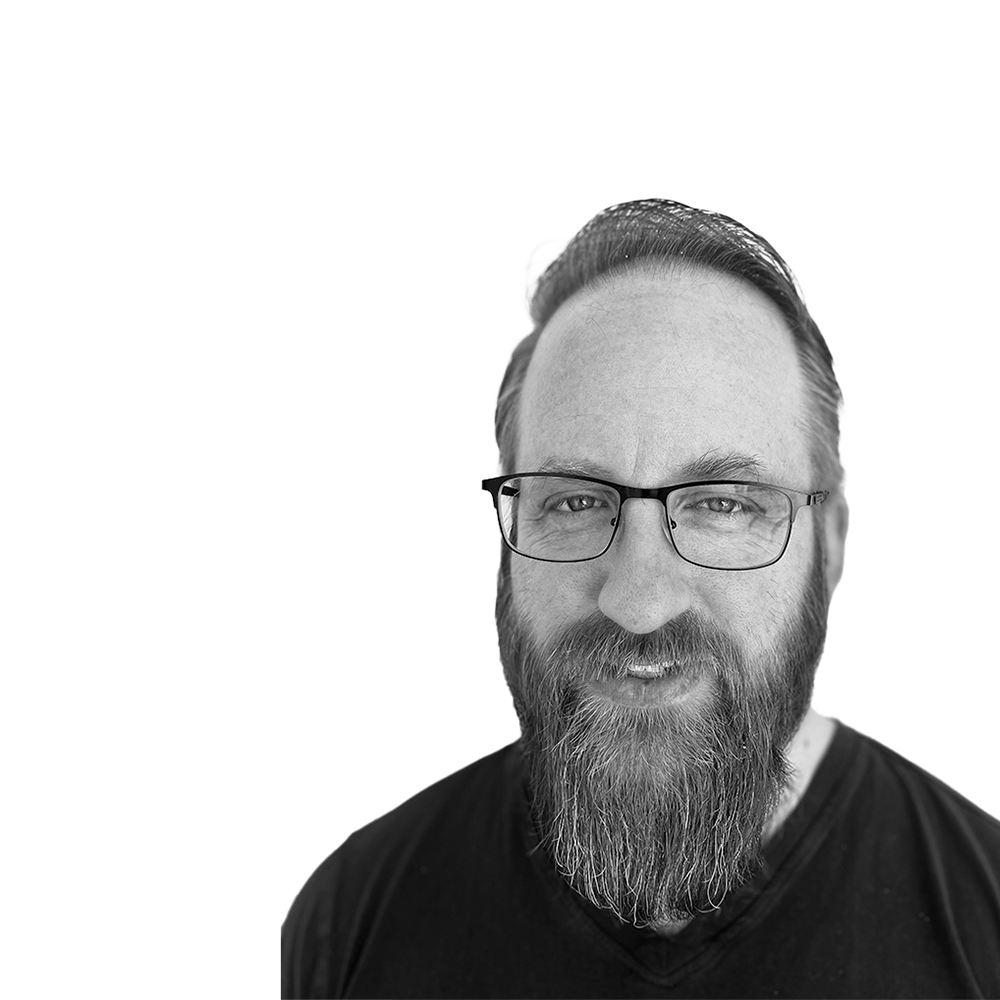
Environmental site assessments (ESA) have long relied on manual data collection, historical research, and expert judgment to identify Areas of Potential Environmental Concern (APECs). However, artificial intelligence (AI) and machine learning (ML) are now revolutionizing the way APECs are identified, mapped, and analyzed—leading to faster, more accurate, and more insightful environmental assessments.
Training AI to Recognize APECs Through Probability Modelling
At Clear-Site AIML Inc., we are integrating machine learning-driven heat mapping to predict the probability of APEC occurrence. By leveraging historically identified APEC data from sites we actively assess, combined with publicly available contaminated site repositories, our AI models can detect contamination trends across industries, geographic regions, and regulatory frameworks.
Using classification-based machine learning techniques, including ID3, CART, Chi-Square, and Reduction algorithms, the system learns to recognize environmental patterns that correlate with contamination sources, migration pathways, and past land uses. This advanced AI training allows for:
- Pattern Recognition in Large Datasets – Identifying recurring contamination sources and risk factors using geospatial and chemical datasets.
- Probability-Based APEC Detection – Generating heat maps that visualize the likelihood of contamination at different site locations.
- Predictive Modeling for Contaminant Spread – Using historical reports to train AI in estimating how APECs evolve over time.
- Automated Report Generation – Streamlining Phase 1 (Stage 1) ESA development by automating background research and identifying key risk areas for investigation.
How ID3, CART, Chi-Square, and Reduction Algorithms Improve APEC Prediction
- ID3 (Iterative Dichotomiser 3): A decision tree algorithm that systematically classifies environmental risk factors based on available data, improving APEC identification accuracy.
- CART (Classification and Regression Trees): A technique that builds predictive models, helping AI determine whether certain site conditions are statistically linked to contamination.
- Chi-Square Algorithm: Evaluates the relationship between independent variables (e.g., soil chemistry, historical land use) and dependent contamination outcomes, refining prediction accuracy.
- Reduction Techniques: Optimize decision trees by removing redundant or insignificant variables, ensuring faster and more reliable APEC mapping.
Adapting AI Through Reinforcement Learning & Thousands of Reports
AI models improve over time through adaptive reinforcement learning, meaning they evolve as they process thousands of environmental reports and regulatory filings. Each new dataset strengthens the model’s ability to:
- Identify subtle contamination patterns missed in traditional assessments.
- Automate repetitive tasks in ESA reporting, reducing time and labor costs.
- Continuously improve accuracy in APEC identification across diverse site conditions.
The Future of AI in Environmental Site Assessments
The ability to train AI models on both proprietary and public datasets means that the future of environmental liability assessment is smarter, faster, and more predictive than ever before. By automating Phase 1 ESA workflows, generating high-accuracy APEC heat maps, and using AI-powered decision trees to refine contamination probability models, Clear-Site Solutions is setting a new industry standard for risk-based liability assessment.
As these systems continue to evolve, they will not only enhance the efficiency of environmental assessments but also provide deeper insights into contamination trends and remediation planning—enabling smarter, data-driven decision-making across the industry.
We're here to help
Want to learn more about our services and how we can help?